class: center, middle, inverse, title-slide # Integrating databases for historical linguistic research ### Peeter Tinits ### DH-Estonia
Tartu, 26.09.2018 --- class: left, middle, inverse ## The question <!-- there is a problem in linguistics --> - Can individuals change language? How much? When? -- - Language tends to live its own life. -- - (e.g. Berg & Aronoff 2017, Amato 2018) -- - Historical sociolinguistics approach -- - Language variation (color / colour) -- - Who did what when to whom, etc, as explanation -- - (e.g. Nevalainen & Raumolin-Brunberg 2012, Auer et al. 2015) -- - Process of language standardization -- - Written Estonian spelling variants ~1880-1920 -- --- class: left ### Historical background - 1880-1920 in Estonia -- - Emerging writing community -- - In transition from a rural to a state language -- - Active effort in language standardization -- - Urbanization, migration between dialects --- class: left ## An emerging community <!--funded by the users etc --> .pull-left2[ 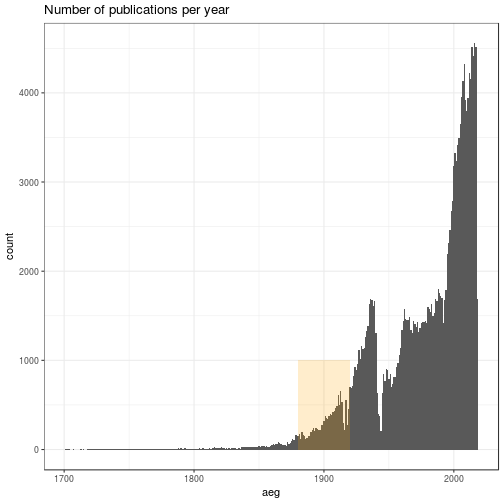<!-- --> ] .pull-right2[ 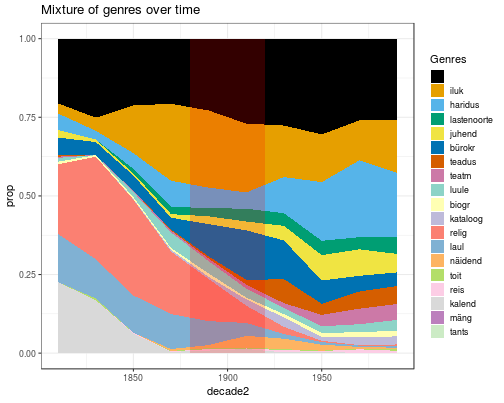<!-- --> ] (data.digar.ee) --- ### Changes in linguistic variation - Why do you use some linguistic form? <!-- (e.g. color/colour) - with some image mb..--> -- - Home dialect? -- - New friends in the city? -- - Traditions? -- - Dictionaries? -- -- - Mechanisms of change -- - Influence of dictionaries/prescription? -- - Who are the early adopters? -- - Population turnover or a change for everyone? -- <!-- [do the older people eventually change or simply stop writing...?]--> --- ## Variation in corpora <!---2 competing forms: e.g. nõu & nõuu [ *advice* ]--->
--- ## A balanced corpus <!--- UT corpus - Snippets from ~100 texts per decade - 1890s, 1900s, 1910s---> 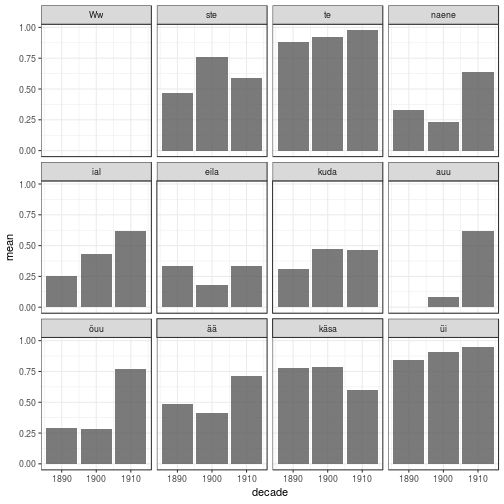<!-- --> --- ## Corpus in detail <!-- so, while balanced corpora are nice, what we'd like in historical sociolinguistics is like this --> <!-- - and when we consider the set of texts, the balancing really does not give us much, - could possibly connect with line vanakorp if author is the same??? -->
--- class: left ## Making use of digitized texts - Gathered available texts (for period 1800-1940) -- - Digar, Literary Museum, E-books, Wikisource, Dspace etc -- - Vary in quality and amount of editing -- - Add metadata (Est. National Bibliography, ISIK biographical database) -- - Usefulness of some text may vary by purpose -- - E.g. OCR not good for general patterns but quite ok for spelling variation -- - On the other hand, edited texts may be unsuitable --- ## Collection overview 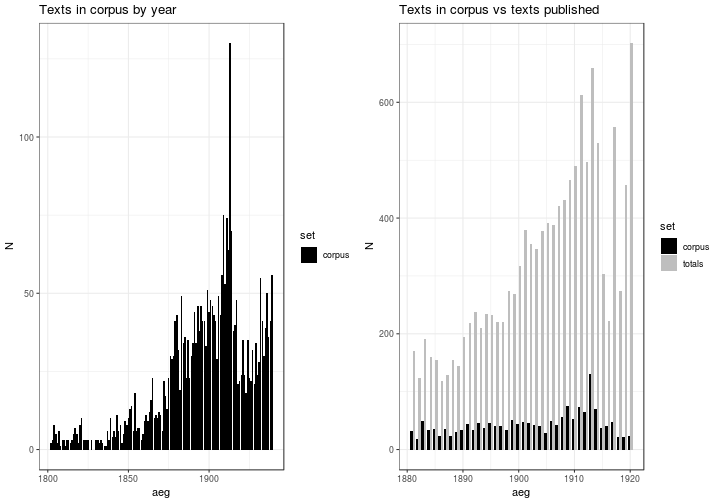<!-- --> --- ## Collection overview 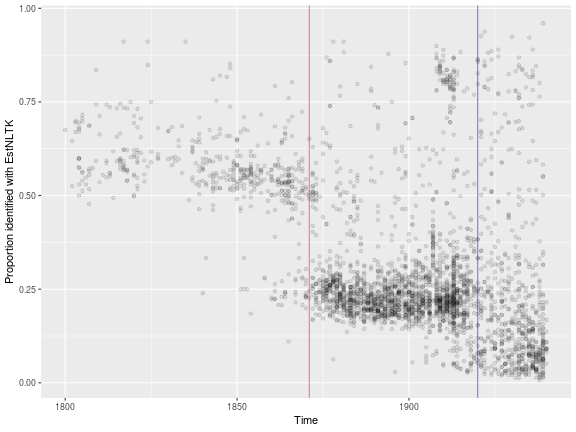<!-- --> --- ## Metainformation on items <img src="data.digar.today.png" width="30%" height="30%" align="middle"> Estonian National Bibliography - publisher, city, date - author, birthdates - print numbers/script, sometimes - genre - topics - etc <!--- years of work, or even generations, when counting the many bibliographies that have been used for it. 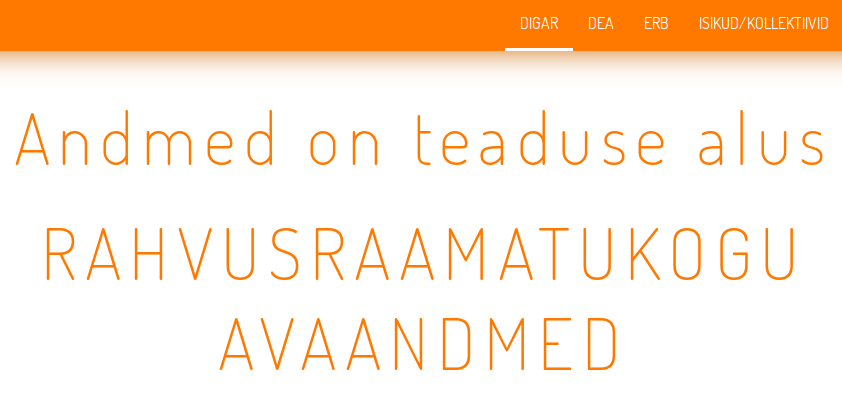{:height="50%" width="50%"} - what is now great though, is that it is added together in one place...--> --- ## Metainformation on items 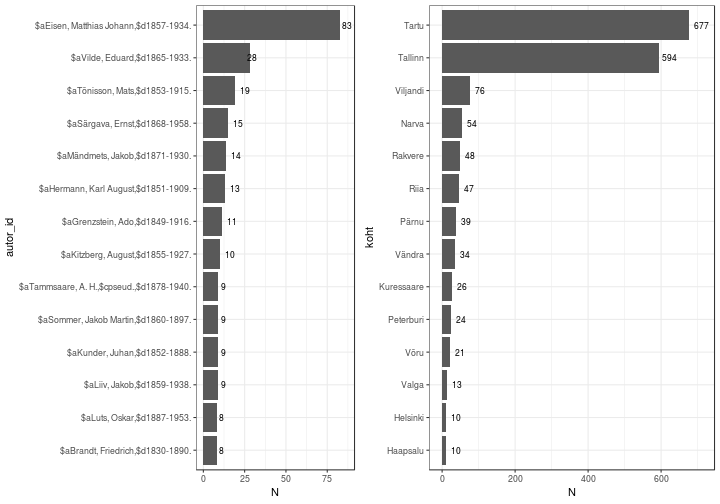<!-- --> --- ## Metainformation on people 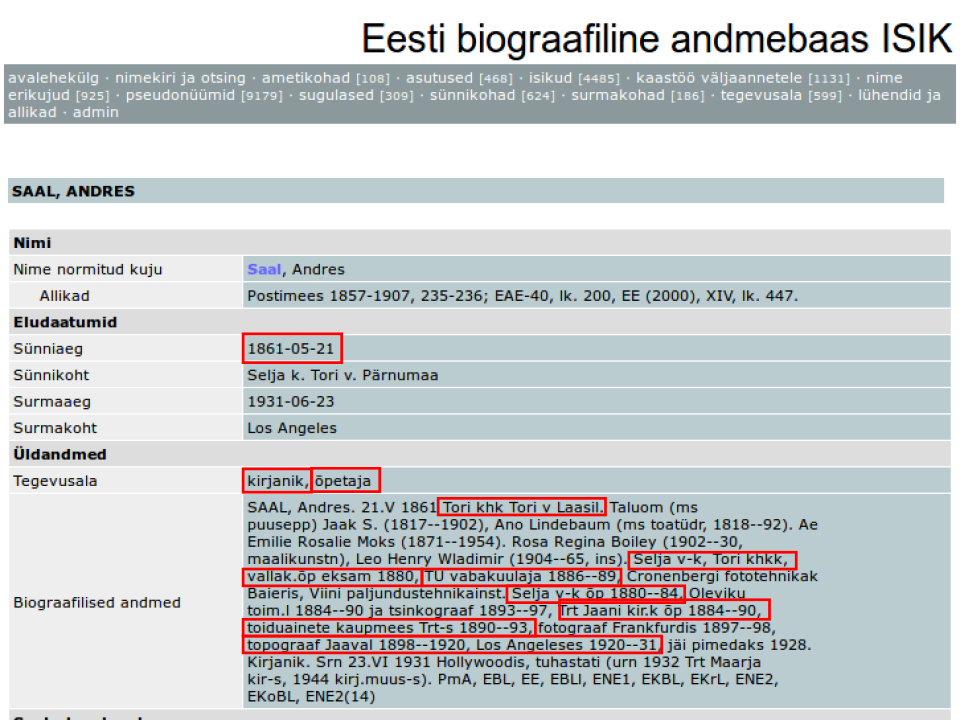 <!--- can make it into list of activities.---> --- ### Placename database 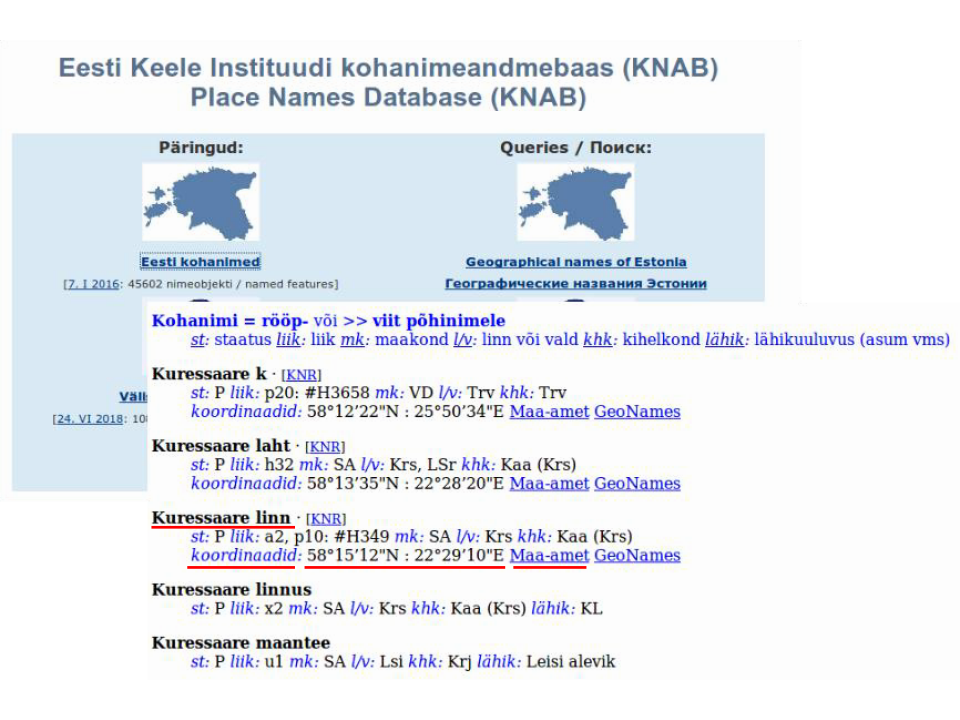 <!--Place name database at Estonian langauge institute,- also has a query system-----> --- ### Merging the information
(Map, Laineste 1997) --- ### Dialect areas
(Uiboaed & Kyrolainen 2015) --- ### Predicting writing from dialect
(Lindström et al. 2015) --- class: inverse, humanities-slide ## Sources on grammars 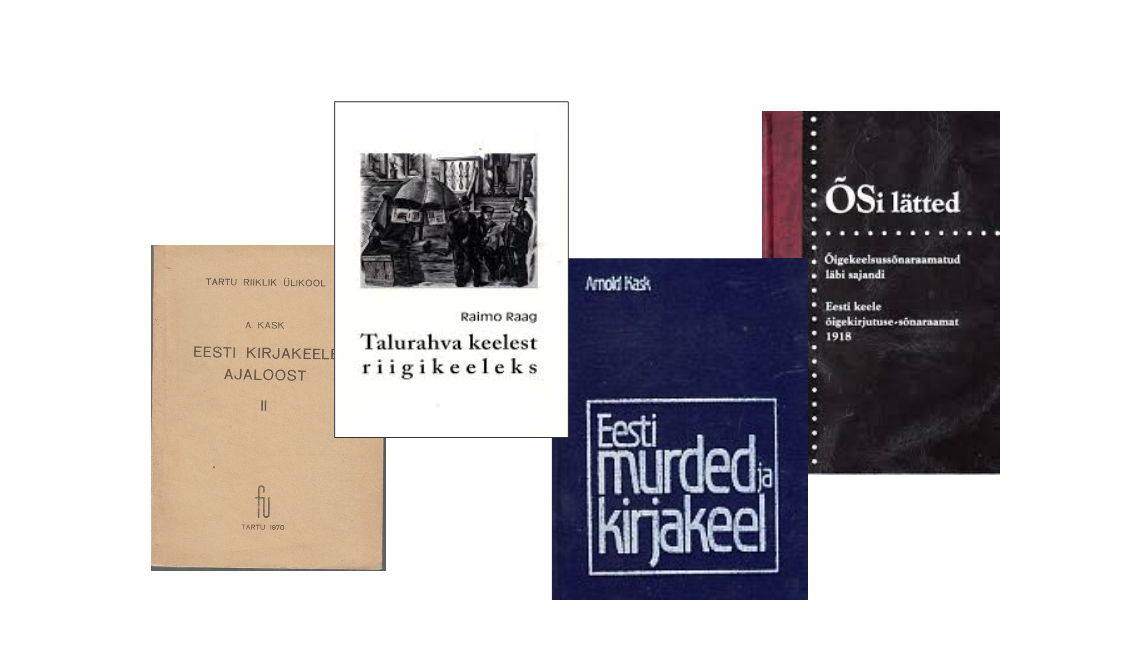 --- ## Corpus results <!---So altogether, referring to the previous available corpus we get a more complete picture, with some nice trends in there... first img of old picture, then img of new picture of averages...---> 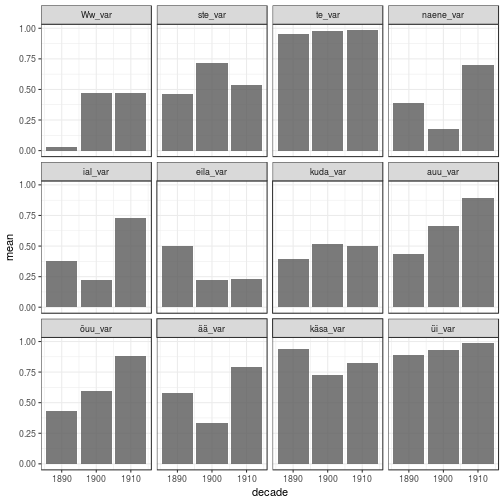<!-- --> --- ## Corpus results 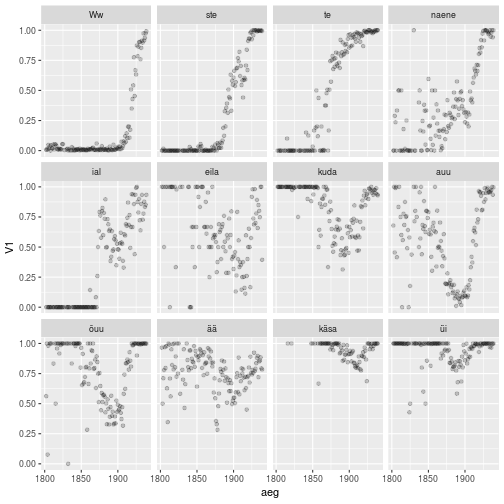<!-- --> --- <!--And in addition to that so we have some nice changes, why did they happen, well the first question we want - can they be associated with intentional activities, picture adding together with prescription,, and indeed it looks like they can, in fact in many occasions these lines are even exact turning points in usage...---> ## Prescription data 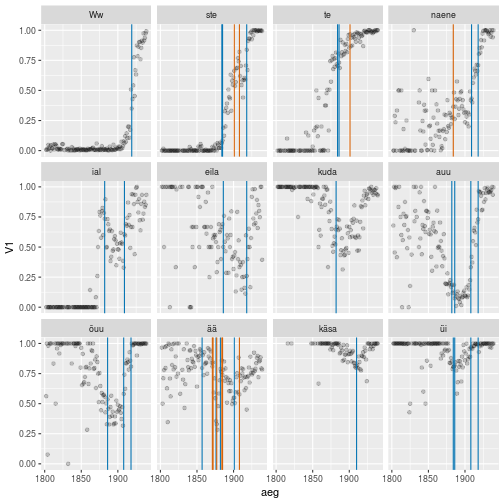<!-- --> --- ## Phases of the change <!--- so, we can zoom in the areas where there seems to be directed changes, like the ones here... and image with trends too maybe?---> 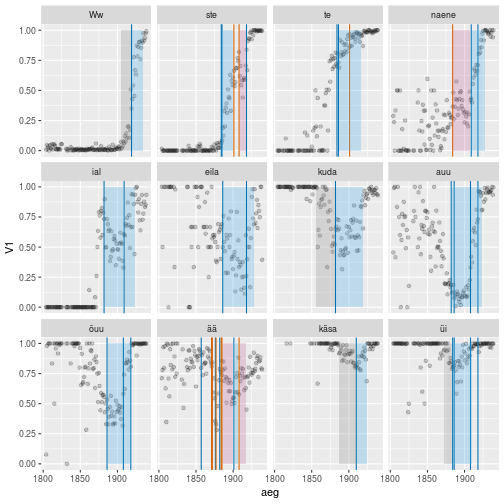<!-- --> --- ### Using the metadata - People - Birthyear - Home dialect - Chosen home dialect - Education level - City size - Publisher - Publisher id - Publisher location - Publisher publications <!---but still, we're not sure who when or under which conditions was doing this... can take a simpler amount of metainformation, the static info about people and publishing.-.. - list - education level (1-5) - chosen domicile, it's dialect background and size - publishing house, location and popsize so while we could use the information where exactly they were living, now we're just keeping it simple...---> --- ### Analysing - So, we want to know the explanations behind individual language choices, and the mechanisms behind language change. -- - What predicts the use of a form? -- - What predicts being ahead or behind of a curve in change? -- - A simple model: Logistic regression to look at determinants of linguistic variation. -- - Considering the trend and grammars: -- - Who were leading the change? -- - Who were following the grammars? <!--we can fit a logistic regression model for each of them, pretty much just characterising the distribution of each of the variables, whether the other dimensions are strongly associated with some linguistic variants? (results preliminary and descriptive...) were the people who used x form likely to be highly educated, living in major cities or young? - can tie back to the hypotheses there again...---> --- ### Note: the data is incomplete <!---now what is important here is that our information is still pretty incomplete, to give an overview we can plot something like this... in time this can become more complete, with more effort and people into it... so with the model we can focus on two broad groups. - for name+date+ location, broadly 1.6k data points depending on the exact phase and variable - should probably put n-s also on the results screen - and for the more detailed metainformatoin set, that includes around 400 data points that have all the required informatoin...---> 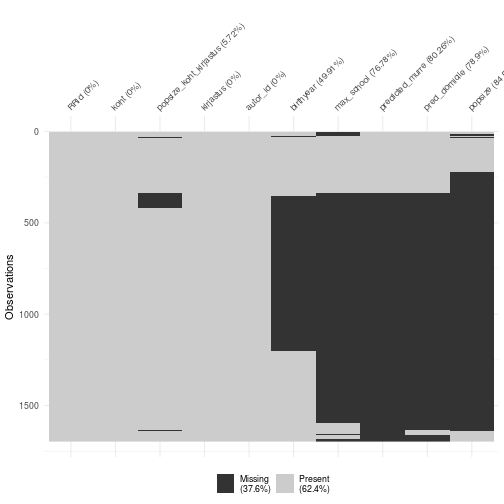<!-- --> --- ### Model results 1 Significant predictors and their directions for a simple model. 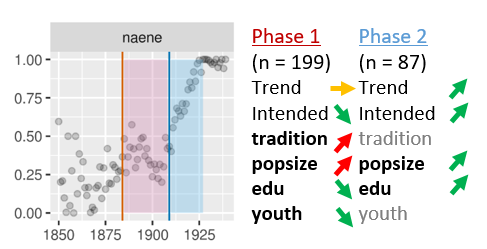 --- ### Model results 1 Significant predictors and their directions for a simple model. 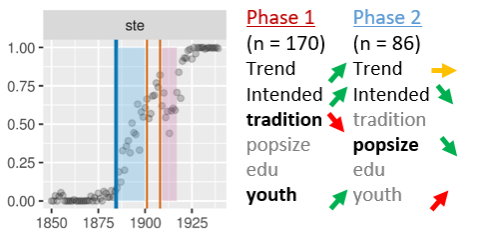 --- ### Model results 2 Significant predictors and their directions for a model with dialect information. 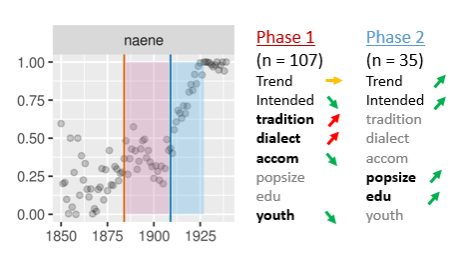 --- ### Model results 2 Significant predictors and their directions for a model with dialect information. 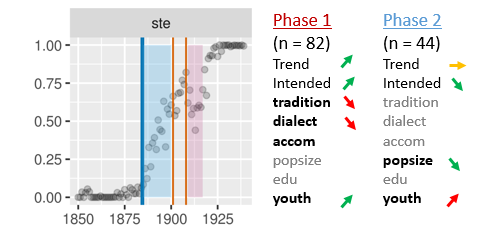 --- ### Model results 3 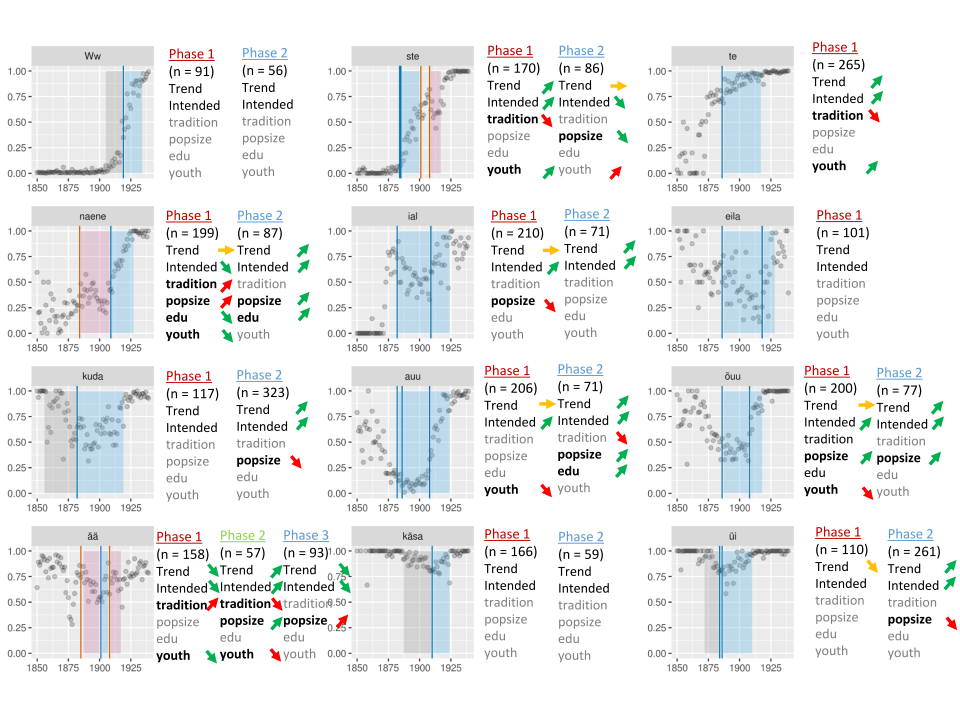 --- ### Model results 4 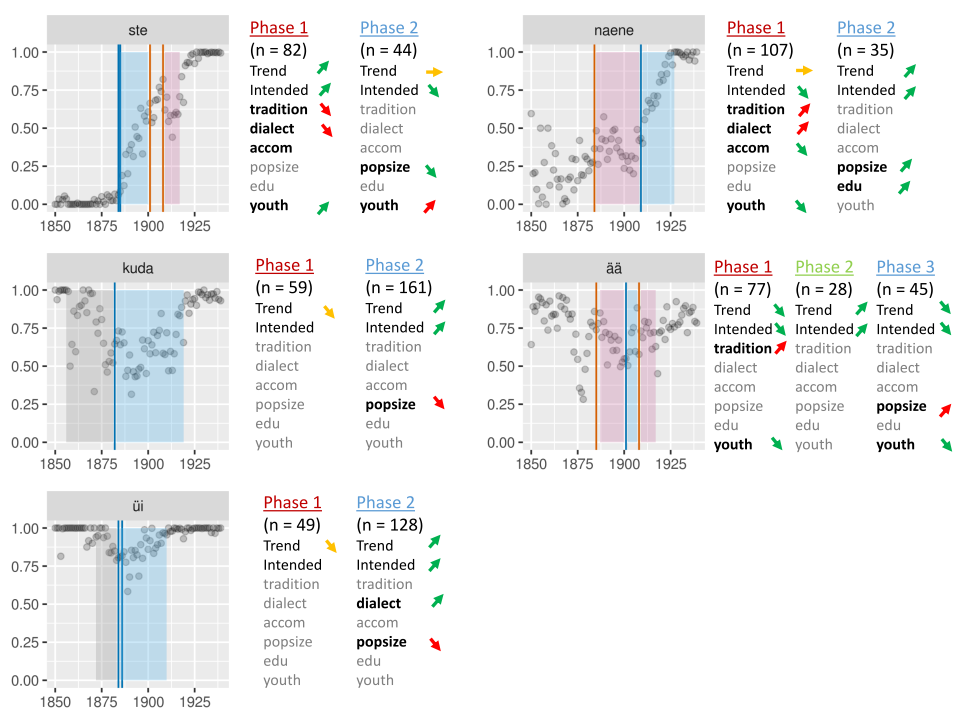 --- ### Model results 5 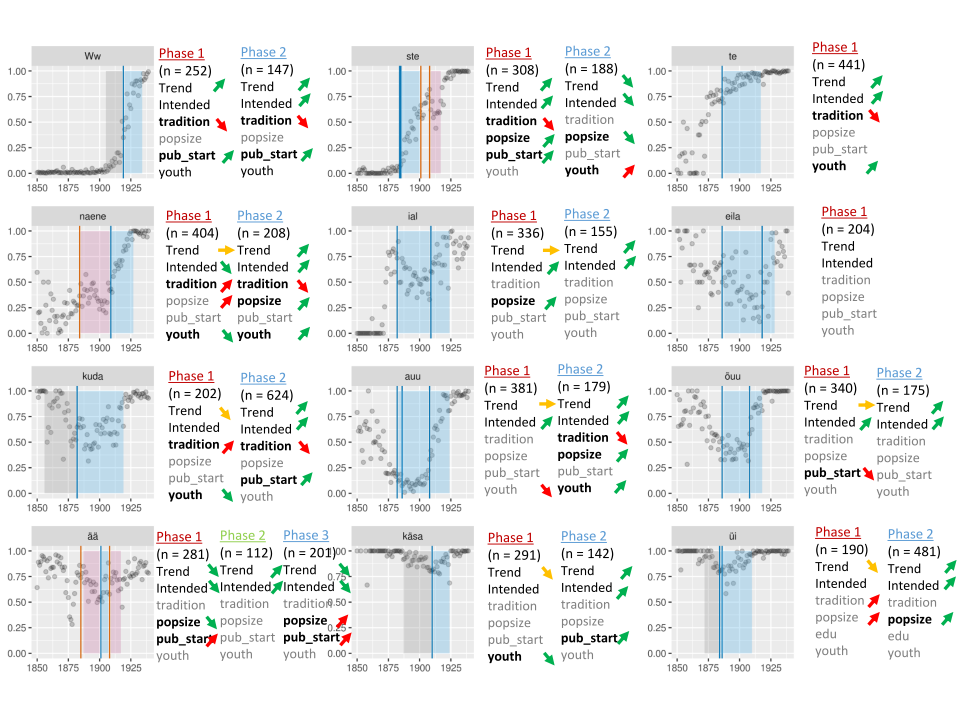 --- ### Model results summary - Simple model results summary 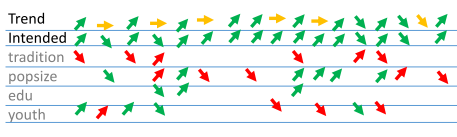 -- - Dialect model results summary 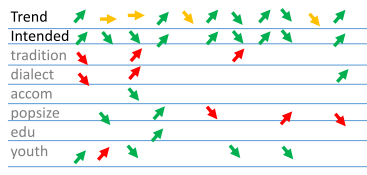 <!---in many phases where it went up or down due to prescription, it was the young people in bigger cities leading the charge, in a few cases it was the opposite.,,, etc---> --- ### What can we conclude? - Some initial inferences - Prescription/grammars influenced language use a lot - Prescription worked always when it was aligned with youth and large cities. - In cases prescription did not work, it had opposition from youth or large cities. - In some cases, prescription also followed existing trends among youths or in cities. - (But this is all a work in progress.) --- ### Combining datasets 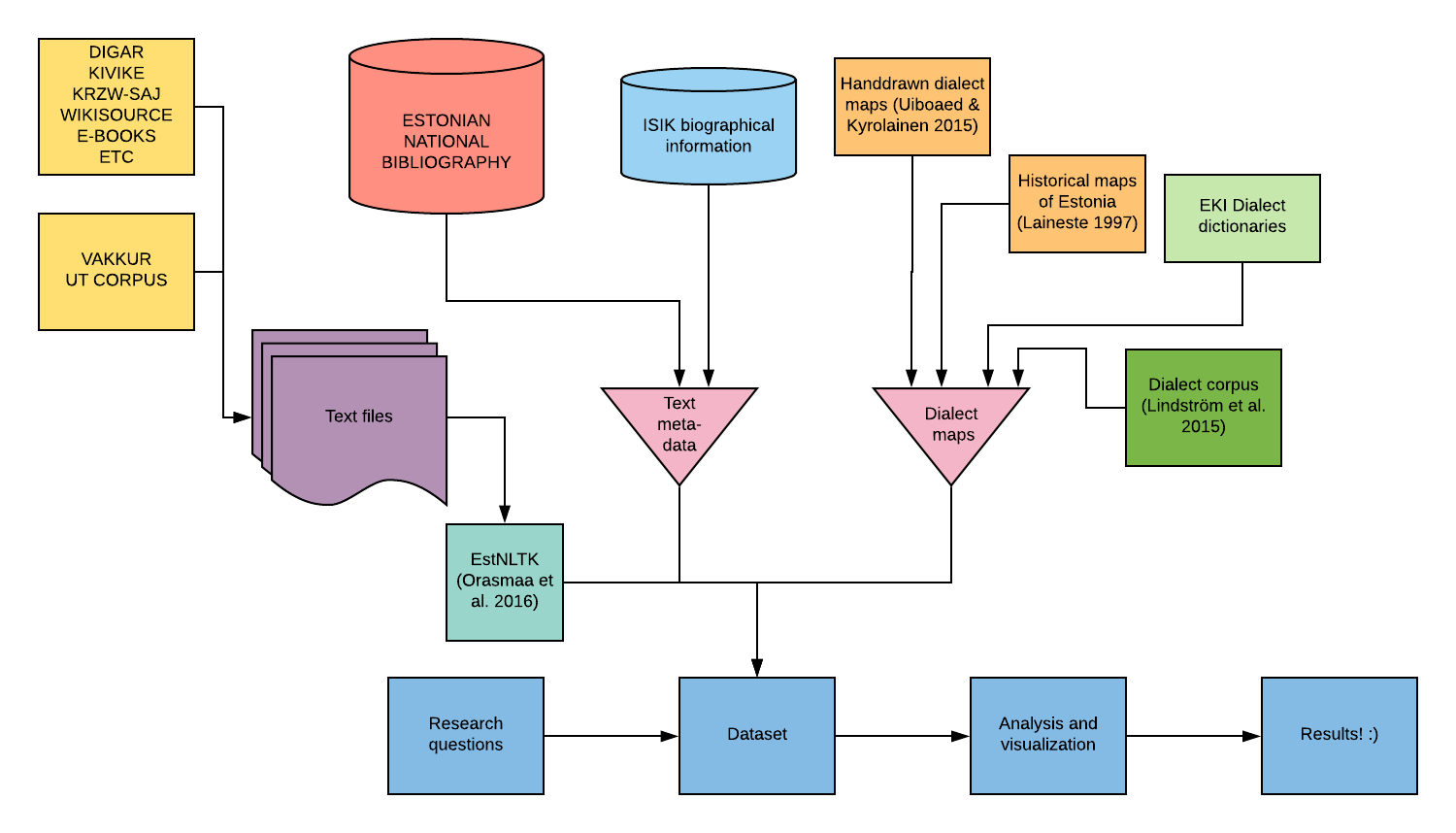 <!--combining metainformatoin with available texts can give pretty interesting results but for this databases need to be compiled and available---> --- ### General summary - Open data allows tackling interesting questions. - There is a lot of unused data available. - Gathering it can be simple if this has option has been kept in mind by maintainers (e.g. ids and links in ENB). - But may depend on half-finished sets and hacks for the others. - As long as they are open they can be reused. ;) <!---particularly, during the project, i have had to rely on a lot of hacks to get into the datasets, as they are often not available, or really meant for that purpose e.g. i really appreciate national libraries data page that wants to be very open and also has active support in how to access it. secondly, the datasets can be put up and available for others too, and to not to keep the good stuff for yourself, using the national bibliography database, we have with the national library gathered the texts up to 1990s that were available into a text corpus (watch out, OCR, but it can be used for many things already...)---> --- class: center, middle, inverse ## Thank you for listening! ### And thank you to all the people who have helped!